Sample size plays a critical role in the accuracy, reliability, and generalizability of analytical results. A larger sample size typically reduces sampling error, increases statistical power, and enhances the precision of estimates. Conversely, a small sample size may lead to unreliable conclusions, increased variability, and a higher risk of Type II errors (failing to detect a true effect). The choice of sample size depends on factors such as the study design, population variability, desired confidence level, and effect size. Properly determining sample size ensures that the analysis is robust and that findings can be confidently applied to the broader population.
Key Points Explained:
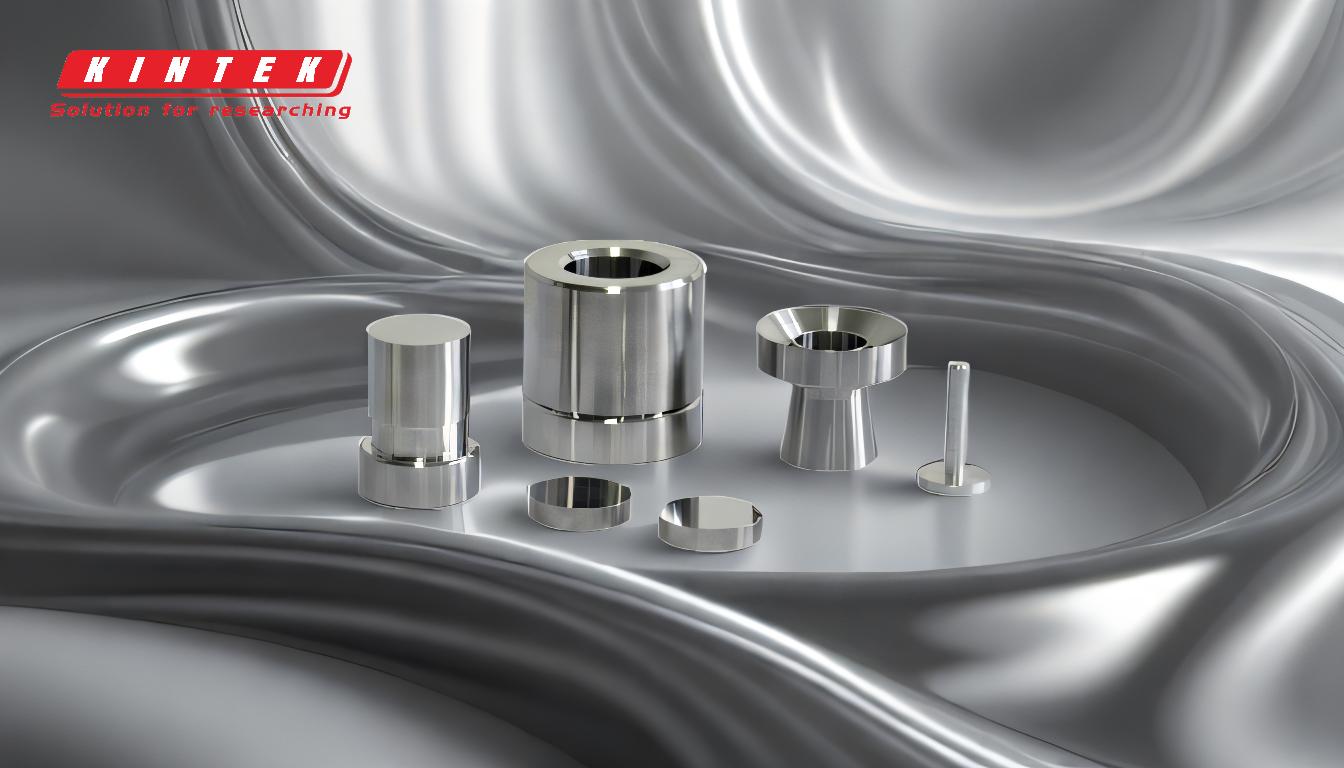
-
Impact on Statistical Power:
- Definition: Statistical power refers to the probability of detecting a true effect when it exists.
- Effect of Sample Size: Larger sample sizes increase statistical power, making it more likely to identify significant effects or differences. For example, in clinical trials, a small sample might miss a treatment's effectiveness, whereas a larger sample would provide more conclusive results.
- Trade-offs: While increasing sample size improves power, it also raises costs and logistical challenges. Researchers must balance these factors to optimize study design.
-
Reduction of Sampling Error:
- Definition: Sampling error is the difference between the sample statistic and the true population parameter.
- Effect of Sample Size: Larger samples reduce sampling error because they better represent the population. For instance, in quality control testing, a small sample might not capture the true variability of a product batch, leading to inaccurate conclusions.
- Practical Implication: In fields like environmental science, where variability is high, larger samples are essential to ensure reliable data.
-
Precision of Estimates:
- Definition: Precision refers to the closeness of repeated measurements or estimates to each other.
- Effect of Sample Size: Larger samples yield narrower confidence intervals, indicating greater precision. For example, in market research, a larger survey sample provides more precise estimates of consumer preferences.
- Application: In manufacturing, precise measurements are critical for ensuring product consistency and meeting quality standards.
-
Generalizability of Results:
- Definition: Generalizability refers to the extent to which findings from a sample can be applied to the broader population.
- Effect of Sample Size: Larger, well-designed samples enhance generalizability by reducing bias and ensuring diversity. For instance, in social science research, a small, non-representative sample might lead to conclusions that do not apply to the entire population.
- Consideration: Researchers must ensure that the sample is not only large but also representative of the population being studied.
-
Risk of Type I and Type II Errors:
-
Definition:
- Type I Error: Incorrectly rejecting a true null hypothesis (false positive).
- Type II Error: Failing to reject a false null hypothesis (false negative).
-
Effect of Sample Size:
- Larger samples reduce the risk of Type II errors by increasing the likelihood of detecting true effects.
- However, very large samples might increase the risk of Type I errors due to heightened sensitivity.
- Example: In pharmaceutical testing, a small sample might fail to detect a drug's side effects, whereas a larger sample would provide more reliable safety data.
-
Definition:
-
Cost and Practical Considerations:
- Definition: The resources required to collect and analyze data, including time, money, and effort.
- Effect of Sample Size: Larger samples demand more resources, which may not always be feasible. Researchers must weigh the benefits of increased accuracy against the costs.
- Solution: Techniques like stratified sampling or power analysis can help optimize sample size without excessive resource expenditure.
-
Ethical Implications:
- Definition: The moral considerations involved in determining sample size, especially in studies involving human or animal subjects.
- Effect of Sample Size: Overly large samples may expose more participants to potential risks without proportional benefits. Conversely, overly small samples may fail to provide meaningful insights, wasting resources and participant involvement.
- Example: In clinical trials, ethical guidelines often require a balance between sufficient sample size for valid results and minimizing participant exposure to experimental treatments.
-
Role of Effect Size:
- Definition: Effect size measures the magnitude of the difference or relationship being studied.
- Effect of Sample Size: Smaller effect sizes require larger samples to detect significant differences. For example, in educational research, detecting small improvements in student performance might necessitate a large sample.
- Application: Understanding effect size helps researchers determine the minimum sample size needed for meaningful analysis.
-
Population Variability:
- Definition: The degree of variation within the population being studied.
- Effect of Sample Size: More variable populations require larger samples to achieve accurate estimates. For instance, in ecological studies, high biodiversity might necessitate larger samples to capture the full range of species and behaviors.
- Consideration: Researchers must account for population variability when planning sample size to avoid biased or incomplete results.
-
Study Design and Sampling Methods:
- Definition: The approach used to select and analyze samples, such as random sampling, stratified sampling, or cluster sampling.
- Effect of Sample Size: The choice of sampling method influences the required sample size. For example, stratified sampling might reduce the needed sample size by ensuring representation across key subgroups.
- Application: In epidemiology, stratified sampling can help ensure that rare conditions or subgroups are adequately represented in the study.
By carefully considering these factors, researchers can determine an appropriate sample size that balances accuracy, reliability, and resource constraints, ultimately leading to more robust and meaningful analyses.
Summary Table:
Factor | Effect of Larger Sample Size | Example |
---|---|---|
Statistical Power | Increases likelihood of detecting true effects | Clinical trials: Larger samples provide conclusive results on treatment efficacy. |
Sampling Error | Reduces error, better represents population | Quality control: Larger samples capture true variability of product batches. |
Precision of Estimates | Yields narrower confidence intervals, indicating greater precision | Market research: Larger surveys provide precise consumer preference data. |
Generalizability | Enhances applicability of findings to the broader population | Social science: Larger, diverse samples ensure conclusions apply widely. |
Type I & II Errors | Reduces risk of Type II errors but may increase Type I errors in very large samples | Pharmaceutical testing: Larger samples detect side effects more reliably. |
Cost & Practicality | Increases resource demands but improves accuracy | Environmental science: Larger samples ensure reliable data despite costs. |
Ethical Implications | Balances participant exposure with meaningful results | Clinical trials: Ethical guidelines ensure sample size is justified. |
Effect Size | Smaller effect sizes require larger samples for detection | Education research: Large samples detect small improvements in performance. |
Population Variability | High variability necessitates larger samples for accurate estimates | Ecology: Larger samples capture biodiversity in variable populations. |
Study Design | Sampling methods influence required sample size | Epidemiology: Stratified sampling ensures rare subgroups are represented. |
Need help determining the right sample size for your research? Contact our experts today for tailored guidance!