X-ray fluorescence (XRF) spectrometry is a powerful analytical tool with numerous advantages, such as fast analysis speed, wide detection range, and non-destructive testing. However, it also has limitations, particularly in its early iterations, which included a narrow detection range and poor sensitivity due to technological constraints. Despite advancements in AI, machine learning, and cloud computing that have improved XRF analysis, certain inherent limitations remain. These include challenges in detecting light elements, matrix effects, and the need for precise calibration. Understanding these limitations is crucial for optimizing the use of XRF in material analysis.
Key Points Explained:
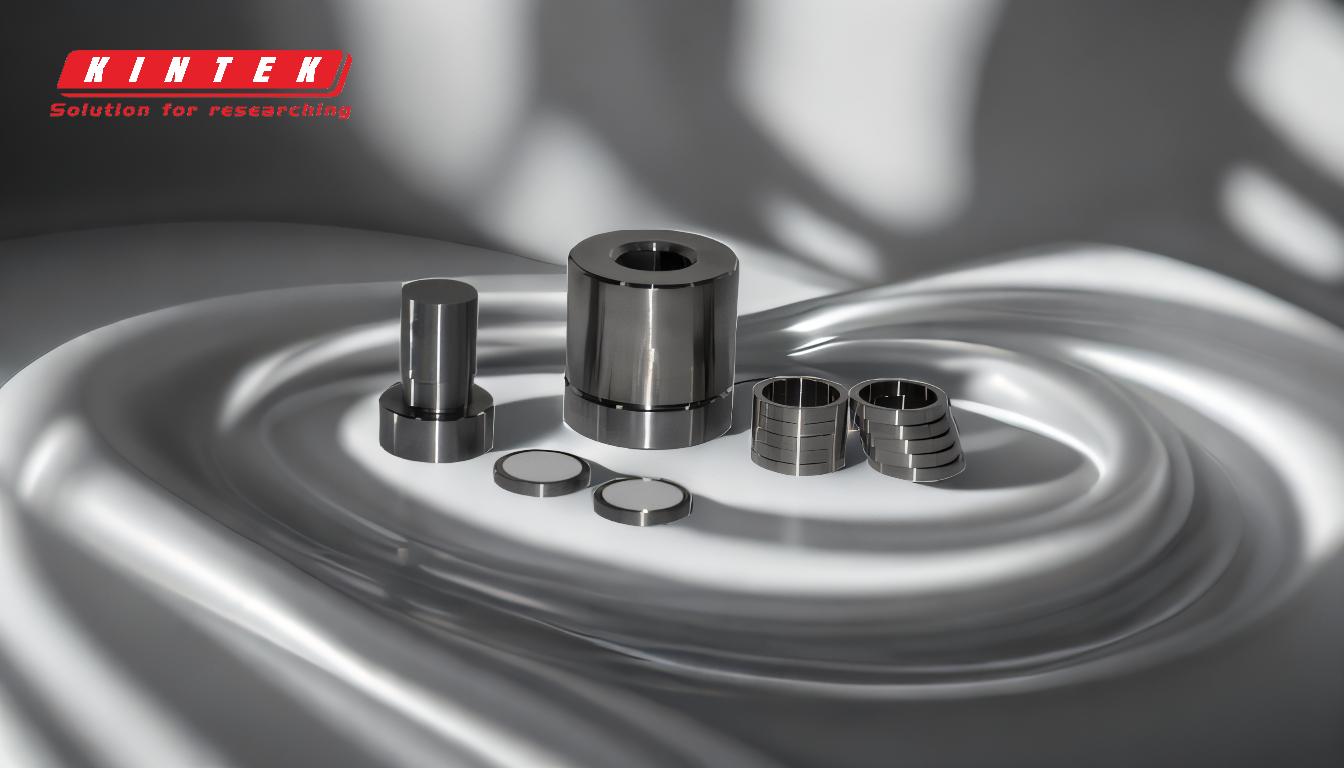
-
Narrow Detection Range and Sensitivity in Early XRF:
- Early XRF spectrometers were limited by the technology of the time, particularly in terms of spectroscopic crystals and detection capabilities. This resulted in a narrow detection range and poor sensitivity, making it difficult to analyze certain materials accurately. While modern XRF systems have improved significantly, these historical limitations highlight the importance of technological advancements in enhancing analytical performance.
-
Challenges in Detecting Light Elements:
- XRF struggles with the detection of light elements (e.g., hydrogen, helium, lithium, and beryllium) because their fluorescence signals are weak and easily absorbed by air or sample matrices. This limitation can affect the accuracy of analyses when light elements are critical components of the material being tested.
-
Matrix Effects:
- Matrix effects occur when the composition of the sample affects the intensity of the X-ray fluorescence signal. For example, elements in a sample can absorb or enhance the fluorescence of other elements, leading to inaccurate results. This requires careful calibration and the use of reference materials to correct for these effects.
-
Need for Precise Calibration:
- XRF analysis relies heavily on calibration to ensure accurate results. Variations in sample composition, surface roughness, and particle size can all affect the calibration. Advanced techniques, such as AI and machine learning, are now being used to improve calibration processes, but the need for precise calibration remains a limitation.
-
Non-Destructive Nature and Its Trade-offs:
- While the non-destructive nature of XRF is a significant advantage, it also limits the depth of analysis. XRF primarily analyzes the surface of a material, and deeper layers may not be accurately represented. This can be a limitation when analyzing heterogeneous materials or when subsurface information is required.
-
Interference and Overlapping Peaks:
- In complex material systems, the fluorescence peaks of different elements can overlap, making it challenging to distinguish between them. This requires sophisticated software and algorithms to deconvolute the spectra, which can still be prone to errors in some cases.
-
Dependence on Sample Preparation:
- Although XRF requires minimal sample preparation compared to other techniques, the quality of the results can still be affected by factors such as sample homogeneity, particle size, and surface condition. Inconsistent sample preparation can lead to variability in results.
-
Technological Advancements Mitigating Limitations:
- Innovations such as AI, machine learning, and cloud computing are addressing some of the limitations of XRF. These technologies enable better calibration, faster data processing, and more accurate analysis, making XRF more versatile and reliable. However, they do not entirely eliminate the inherent limitations of the technique.
In summary, while XRF is a highly effective analytical tool with many advantages, it is not without limitations. Understanding these limitations and leveraging technological advancements can help users optimize their XRF analyses and achieve more accurate and reliable results.
Summary Table:
Limitation | Description |
---|---|
Narrow Detection Range | Early XRF systems had limited detection capabilities, affecting accuracy. |
Light Element Detection | Weak fluorescence signals make detecting hydrogen, helium, etc., challenging. |
Matrix Effects | Sample composition can alter fluorescence signals, requiring careful calibration. |
Precise Calibration | Variations in sample properties necessitate advanced calibration techniques. |
Non-Destructive Trade-offs | Surface analysis limits depth insights for heterogeneous materials. |
Interference and Overlapping Peaks | Overlapping element signals complicate analysis. |
Sample Preparation Dependence | Inconsistent preparation can lead to variable results. |
Technological Advancements | AI and machine learning improve calibration but don’t eliminate limitations. |
Need help optimizing your XRF analysis? Contact our experts today for tailored solutions!